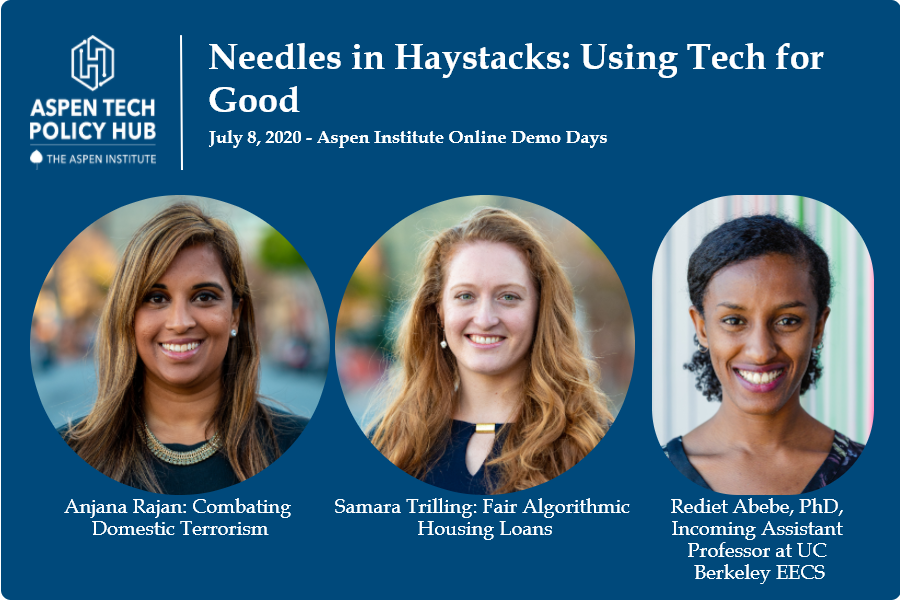
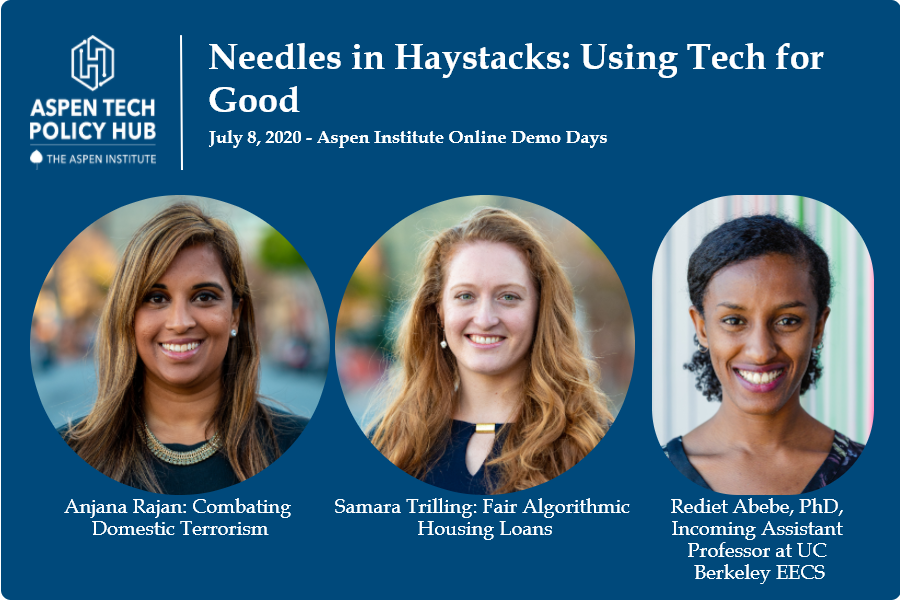
San Francisco, CA – It is our pleasure to introduce the second set of projects from our 2020 Fellowship class.
The projects, focused on Needles in Haystacks: Using Tech for Good, provide exciting solutions for combating domestic terrorism and algorithmic fairness in mortgage lending decisions. We released the projects at our webinar today; you can watch the video of the event here.
We invite you to read more about the projects below, and to check them out on our website: Aspen Tech Policy Hub Projects. Please also join us by RSVPing for our next webinar, July 22 at 9am PT/12pm ET, on Seeding Success: Equity, Technology & Policy, focused on EdTech Equity, Closing the Tech Funding Gap, and People Powered Policy.
In order to thwart mass gun violence caused by white supremacy extremists, witnesses must take action. An FBI study on active shooters shows that there were on average three distinct witnesses who observed concerning behaviors about the suspect prior to their attack. Unfortunately, nearly 60 percent of witnesses did not report to the police, resulting in many missed opportunities to save lives. This low reporting rate can be solved through the use of a digital reporting escrow. Witnesses can report concerning behaviors into an escrow and their report would stay locked until a credible threshold of risk was met. This system motivates witnesses to report suspicious behavior while protecting the civil liberties of the accused.
Mortgage lenders increasingly use machine learning (ML) algorithms to make loan approval and pricing decisions. This has some positive effects: ML loan models can be up to 40 percent less discriminatory than face-to-face lending. Moreover, unlike human loan officers, algorithms can be tested for fairness before they’re released into the wild. But such decisions also present challenges: when ML models discriminate, they do so disproportionately against underbanked borrowers. In addition, it is often unclear how existing fair lending laws should be applied to algorithms, and they are updated too frequently for traditional fair lending audits to handle. To address these challenges, this project recommends that New York State banking regulators define a fairness metric for mortgage algorithms and pilot automated fair lending tests.
Learn more about these and other projects here.